Analyzing Images
Once an image is submitted to Anchore Enterprise for analysis, Anchore Enterprise will attempt to retrieve metadata about the image from the Docker registry and, if successful, will download the image and queue the image for analysis.
Anchore Enterprise can run one or more analyzer services to scale out processing of images. The next available analyzer worker will process the image.
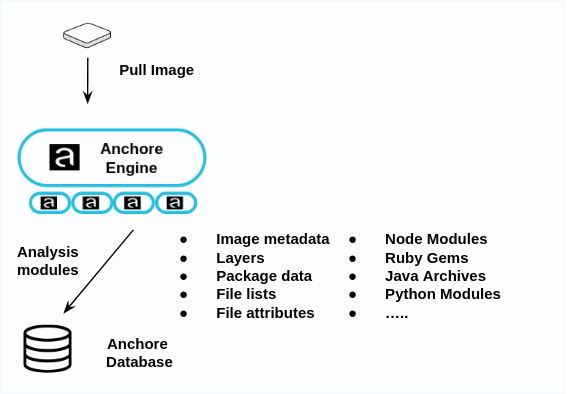
During analysis, every package, software library, and file are inspected, and this data is stored in the Anchore database.
Anchore Enterprise includes a number of analyzer modules that extract data from the image including:
- Image metadata
- Image layers
- Operating System Package Data (RPM, DEB, APKG)
- File Data
- Ruby Gems
- Node.JS NPMs
- Java Archives
- Python Packages
- .NET NuGet Packages
- File content
Once a tag has been added to Anchore Enterprise, the repository will be monitored for updates to that tag. See Image and Tag Watchers for more information about images and tags.
Any updated images will be downloaded and analyzed.
Next Steps
Now let’s get familiar with the Image Analysis Process.
1 - Base and Parent Images
A Docker or OCI image is composed of layers. Some of the layers are created during a build process such as following instructions in a Dockerfile. But many of the layers will come from previously built images. These images likely come from a container team at your organization, or maybe build directly on images from a Linux distribution vendor. In some cases this chain could be many images deep as various teams add standard software or configuration.
Docker uses the FROM
clause to denote an image to use as a basis for building a new image. The image provided in this clause is known by Docker as the Parent Image, but is commonly referred to as the Base Image. This chain of images built from other images using the FROM
clause is known as an Image’s ancestry.
Note Docker defines Base Image as an image with a FROM SCRATCH
clause. Anchore does NOT follow this definition, instead following the more common usage where Base Image refers to the image that a given image was built from.
Example Ancestry
The following is an example of an image with multiple ancestors
A base distro image, for example debian:10
FROM scratch
...
A framework container image from that debian image, for example a node.js image let’s call mynode:latest
FROM debian:10
# Install nodejs
The application image itself built from the framework container, let’s call it myapp:v1
FROM mynode:latest
COPY ./app /
...
These dockerfiles generate the following ancestry graph:
graph
debian:10-->|parent of|mynode:latest
mynode:latest-->|parent of|myapp:v1
Where debian:10
is the parent of mynode:latest
which is the parent of myapp:v1
Anchore compares the layer digests of images that it knows about to determine an images ancestry. This ensures that the exact image used to build a new image is identified.
Given our above example, we may see the following layers for each image. Note that each subsequent image is a superset of the previous images layers
block-beta
columns 5
block:debian_block
columns 1
debian["debian:10"]
style debian stroke-width:0px
debian_layer1["sha256:abc"]
debian_layer2["sha256:def"]
space
space
end
space
block:mynode_block
columns 1
mynode_name["mynode:latest"]
style mynode_name stroke-width:0px
mynode_layer1["sha256:abc"]
mynode_layer2["sha256:def"]
mynode_layer3["sha256:123"]
space
end
space
block:myapp_block
columns 1
myapp_name["myapp:v1"]
style myapp_name stroke-width:0px
myapp_layer1["sha256:abc"]
myapp_layer2["sha256:def"]
myapp_layer3["sha256:123"]
myapp_layer4["sha256:456"]
end
mynode_name -- "FROM" --> debian
debian_layer1 --> mynode_layer1
mynode_layer1 --> debian_layer1
debian_layer2 --> mynode_layer2
mynode_layer2 --> debian_layer2
myapp_name -- "FROM" --> mynode_name
mynode_layer1 --> myapp_layer1
myapp_layer1 --> mynode_layer1
mynode_layer2 --> myapp_layer2
myapp_layer2 --> mynode_layer2
mynode_layer3 --> myapp_layer3
myapp_layer3 --> mynode_layer3
Ancestry within Anchore
Anchore automatically calculates an image’s ancestry as images are scanned. This works by comparing the layer digests of each image to calculate the entire chain of images that produced a given image. The entire ancestry can be retrieved for an image through the GET /v2/images/{image_digest}/ancestors
API. See the API docs for more information on the specifics.
Base Image
It is often useful to compare an image with another image in its ancestry. For example to filter out vulnerabilities that are present in a “golden image” from a platform team and only showing vulnerabilities introduced by the application being built on the “golden image”.
Controlling the Base Image
Users can control which ancestor is chosen as the base image by marking the desired image(s) with a special annotation anchore.user/marked_base_image
. The annotation should be set to a value of true
, otherwise it will be ignored. This annotation is currently restricted to users in the “admin” account.
If an image with this annotation should no longer be considered a Base Image than you must update the annotation to false
, as it is not currently possible to remove annotations.
Usage of this annotation when calculating the Base Image can be disabled by setting services.policy_engine.enable_user_base_image
to false in the configuration file (see deployment specific docs for configuring this setting).
Anchorectl Example
You can add an image with this annotation using AnchoreCTL with the following:
anchorectl image add anchore/test_images:ancestor-base -w --annotation "anchore.user/marked_base_image=true"
If an image should no longer be considered a Base Image you can update the annotation with:
anchorectl image add anchore/test_images:ancestor-base --annotation "anchore.user/marked_base_image=false"
Calculating the Base Image
Anchore will automatically calculate the Base Image from an image’s ancestry using the closest ancestor. From our example above, the Base Image for myapp:v1
is mynode:latest
.
The first ancestor with this annotation will be used as the Base Image, if no ancestors have this annotation than it will fall back to using the closest ancestor (the Parent Image).
The rules for determining the Base Image are encoded in this diagram
graph
start([start])-->image
image[image]
image-->first_parent_exists
first_parent_exists{Does this image have a parent?}
first_parent_exists-->|No|no_base_image
first_parent_exists-->|yes|first_parent_image
first_parent_image[Parent Image]
first_parent_image-->config
config{User Base Annotations Enabled in configuration?}
config-->|No|base_image
config-->|yes|check_parent
check_parent{Parent has anchore.user/marked_base_image: true annotation}
check_parent-->|No|parent_exists
parent_exists{Does the parent image have a parent?}
parent_exists-->|Yes|parent_image
parent_image[/Move to next Parent Image/]
parent_image-->check_parent
parent_exists-->|No|no_base_image
check_parent-->|Yes|base_image
base_image([Found Base Image])
no_base_image([No Base Image Exists])
Using the Base Image
The Policy evaluation and Vuln Scan APIs have an optional base_digest
parameter that is used to provide comparison data between two images. These APIs can be used in conjunction with the ancestry API to perform comparisons to the Base Image so that application developers can focus on results in their direct control. As of Enterprise v5.7.0, a special value auto
can also be specified for this parameter to have the system automatically determine which image to use in the comparison based on the above rules.
To read more about the base comparison features, jump to
In addition to these user facing APIs, a few parts of the system utilize the Ancestry information.
- The Ancestry Policy Gate uses the Base Image rules to determine which image to evaluate against
- Reporting uses the Base Image to calculate the “Inherited From Base” column for vulnerabilities
- The UI displays the Base Image and uses it for Policy Evaluations and Vulnerability Scans
Additional notes about ancestor calculations
An image B is only a child of Image A if All of the layers of Image A are present in Image B.
For example, mypython
and mynode
represent two different language runtime images built from a debian base. These two images are not ancestors of each other because the layers in mypython:latest
are not a superset of the layers in mynode:latest
, nor the other way around.
block-beta
columns 3
block:mypython_block
columns 1
mypython_name["mypython:latest"]
style mypython_name stroke-width:0px
mypython_layer1["sha256:abc"]
mypython_layer2["sha256:def"]
mypython_layer3["sha256:456"]
end
space
block:mynode_block
columns 1
mynode_name["mynode:latest"]
style mynode_name stroke-width:0px
mynode_layer1["sha256:abc"]
mynode_layer2["sha256:def"]
mynode_layer3["sha256:123"]
end
mypython_layer1 --> mynode_layer1
mynode_layer1 --> mypython_layer1
mypython_layer2 --> mynode_layer2
mynode_layer2 --> mypython_layer2
mypython_layer3 -- "X" --> mynode_layer3
But these images could be based on a 3rd image which is made up of the 2 layers that they share. If Anchore knows about this 3rd image it would show up as an ancestor for both mypython
and mynode
.
The Anchore UI would identify the debian:10
image as an ancestor of the mypython:latest
and the mynode:latest
images. But we do not currently expose child ancestors, so we would not show the children of the debian:10
image.
block-beta
columns 5
block:mypython_block
columns 1
mypython_name["mypython:latest"]
style mypython_name stroke-width:0px
mypython_layer1["sha256:abc"]
mypython_layer2["sha256:def"]
mypython_layer4["sha256:456"]
end
space
block:debian_block
columns 1
debian["debian:10"]
style debian stroke-width:0px
debian_layer1["sha256:abc"]
debian_layer2["sha256:def"]
space
end
space
block:mynode_block
columns 1
mynode_name["mynode:latest"]
style mynode_name stroke-width:0px
mynode_layer1["sha256:abc"]
mynode_layer2["sha256:def"]
mynode_layer3["sha256:123"]
end
mynode_name -- "FROM" --> debian
debian_layer1 --> mynode_layer1
mynode_layer1 --> debian_layer1
debian_layer2 --> mynode_layer2
mynode_layer2 --> debian_layer2
mypython_name -- "FROM" --> debian
debian_layer1 --> mypython_layer1
mypython_layer1 --> debian_layer1
debian_layer2 --> mypython_layer2
mypython_layer2 --> debian_layer2
1.1 - Compare Base Image Policy Checks
This feature provides a mechanism to compare the policy checks for an image with those of a Base Image. You can read more about Base Image and how to
find them here. Base comparison uses the same policy and tag to evaluate both images to
ensure a fair comparison. The API yields a response similar to the policy checks API with an additional element within each triggered gate check to
indicate whether the result is inherited from the Base Image.
Usage
This functionality is currently available via the Enterprise UI and API.
API
Refer to API Access section for the API specification. The policy check API (GET /v2/images/{imageDigest}/check
) has
an optional base_digest
query parameter that can be used to specify an image to compare policy findings to. When this query parameter is provided
each of the finding’s inherited_from_base
field will be filled in with true
or false
to denote if the finding is present in the provided image.
If no image is provided than the inherited_from_base
field will be null
to indicate no comparison was performed.
Example request using curl to retrieve policy check for an image digest sha256:xyz
and tag p/q:r
and compare the results to a Base Image digest sha256:abc
curl -X GET -u {username:password} "http://{servername:port}/v2/images/sha256:xyz/check?tag=p/q:r&base_digest=sha256:abc"
Example output:
{
"image_digest": "sha256:xyz",
"evaluated_tag": "p/q:r",
"evaluations": [
{
"comparison_image_digest": "sha256:abc",
"details": {
"findings": [
{
"trigger_id": "41cb7cdf04850e33a11f80c42bf660b3",
"gate": "dockerfile",
"trigger": "instruction",
"message": "Dockerfile directive 'HEALTHCHECK' not found, matching condition 'not_exists' check",
"action": "warn",
"policy_id": "48e6f7d6-1765-11e8-b5f9-8b6f228548b6",
"recommendation": "",
"rule_id": "312d9e41-1c05-4e2f-ad89-b7d34b0855bb",
"allowlisted": false,
"allowlist_match": null,
"inherited_from_base": true
},
{
"trigger_id": "CVE-2019-5435+curl",
"gate": "vulnerabilities",
"trigger": "package",
"message": "MEDIUM Vulnerability found in os package type (APKG) - curl (CVE-2019-5435 - http://cve.mitre.org/cgi-bin/cvename.cgi?name=CVE-2019-5435)",
"action": "warn",
"policy_id": "48e6f7d6-1765-11e8-b5f9-8b6f228548b6",
"recommendation": "",
"rule_id": "6b5c14e7-a6f7-48cc-99d2-959273a2c6fa",
"allowlisted": false,
"allowlist_match": null,
"inherited_from_base": false
}
]
...
}
...
}
...
]
}
Dockerfile directive 'HEALTHCHECK' not found, matching condition 'not_exists' check
is triggered by both images and hence inherited_from_base
is marked true
MEDIUM Vulnerability found in os package type (APKG) - curl (CVE-2019-5435 - http://cve.mitre.org/cgi-bin/cvename.cgi?name=CVE-2019-5435)
is not
triggered by the Base Image and therefore the value of inherited_from_base
is false
1.2 - Compare Base Image Security Vulnerabilities
This feature provides a mechanism to compare the security vulnerabilities detected in an image with those of a Base Image. You can read more about base
images and how to find them here. The API yields a response similar to vulnerabilities API with an
additional element within each result to indicate whether the result is inherited from the Base Image.
Usage
This functionality is currently available via the Enterprise UI and API. Watch this space as we add base comparison support in other tools.
API
Refer to API Access section for the API specification. The vulnerabilities API GET /v2/images/{image_digest}/vuln/{vtype}
has a base_digest
query parameter that can be used to specify an image to compare vulnerability findings to. When this query parameter is provided
an additional inherited_from_base
field is provided for each vulnerability.
Example request using curl to retrieve security vulnerabilities for an image digest sha:xyz
and compare the results to a Base Image digest sha256:abc
curl -X GET -u {username:password} "http://{servername:port}/v2/images/sha256:xyz/vuln/all?base_digest=sha256:abc"
Example output:
{
"base_digest": "sha256:abc",
"image_digest": "sha256:xyz",
"vulnerability_type": "all",
"vulnerabilities": [
{
"feed": "vulnerabilities",
"feed_group": "alpine:3.12",
"fix": "7.62.0-r0",
"inherited_from_base": true,
"nvd_data": [
{
"cvss_v2": {
"base_score": 6.4,
"exploitability_score": 10.0,
"impact_score": 4.9
},
"cvss_v3": {
"base_score": 9.1,
"exploitability_score": 3.9,
"impact_score": 5.2
},
"id": "CVE-2018-16842"
}
],
"package": "libcurl-7.61.1-r3",
"package_cpe": "None",
"package_cpe23": "None",
"package_name": "libcurl",
"package_path": "pkgdb",
"package_type": "APKG",
"package_version": "7.61.1-r3",
"severity": "Medium",
"url": "http://cve.mitre.org/cgi-bin/cvename.cgi?name=CVE-2018-16842",
"vendor_data": [],
"vuln": "CVE-2018-16842"
},
{
"feed": "vulnerabilities",
"feed_group": "alpine:3.12",
"fix": "2.4.46-r0",
"inherited_from_base": false,
"nvd_data": [
{
"cvss_v2": {
"base_score": 5.0,
"exploitability_score": 10.0,
"impact_score": 2.9
},
"cvss_v3": {
"base_score": 7.5,
"exploitability_score": 3.9,
"impact_score": 3.6
},
"id": "CVE-2020-9490"
}
],
"package": "apache2-2.4.43-r0",
"package_cpe": "None",
"package_cpe23": "None",
"package_name": "apache2",
"package_path": "pkgdb",
"package_type": "APKG",
"package_version": "2.4.43-r0",
"severity": "Medium",
"url": "http://cve.mitre.org/cgi-bin/cvename.cgi?name=CVE-2020-9490",
"vendor_data": [],
"vuln": "CVE-2020-9490"
}
]
}
Note that inherited_from_base
is a new element in the API response added to support base comparison. The assigned boolean value indicates whether the
exact vulnerability is present in the Base Image. In the above example
- CVE-2018-16842 affects libcurl-7.61.1-r3 package in both images, hence
inherited_from_base
is marked true
- CVE-2019-5482 affects apache2-2.4.43-r0 package does not affect the Base Image and therefore
inherited_from_base
is set to false
2 - Image Analysis Process
There are two types of image analysis:
- Centralized Analysis
- Distributed Analysis
Image analysis is performed as a distinct, asynchronous, and scheduled
task driven by queues that analyzer workers periodically poll.
Image analysis_status
states:
stateDiagram
[*] --> not_analyzed: analysis queued
not_analyzed --> analyzing: analyzer starts processing
analyzing --> analyzed: analysis completed successfully
analyzing --> analysis_failed: analysis fails
analyzing --> not_analyzed: re-queue by timeout or analyzer shutdown
analysis_failed --> not_analyzed: re-queued by user request
analyzed --> not_analyzed: re-queued for re-processing by user request
Centralized Analysis
The analysis process is composed of several steps and utilizes several
system components. The basic flow of that task as shown in the following example:
Centralized analysis high level summary:
sequenceDiagram
participant A as AnchoreCTL
participant R as Registry
participant E as Anchore Deployment
A->>E: Request Image Analysis
E->>R: Get Image content
R-->>E: Image Content
E->>E: Analyze Image Content (Generate SBOM and secret scans etc) and store results
E->>E: Scan sbom for vulns and evaluate compliance
The analyzers operate in a task loop for analysis tasks as shown below:

Adding more detail, the API call trace between services looks similar to the following example flow:

Distributed Analysis
In distributed analysis, the analysis of image content takes place outside the Anchore deployment and the result is imported
into the deployment. The image has the same state machine transitions, but the ‘analyzing’ processing of an imported analysis
is the processing of the import data (vuln scanning, policy checks, etc) to prepare the data for internal use, but does not download or touch any image content.
High level example with AnchoreCTL:
sequenceDiagram
participant A as AnchoreCTL
participant R as Registry/Docker Daemon
participant E as Anchore Deployment
A->>R: Get Image content
R-->>A: Image Content
A->>A: Analyze Image Content (Generate SBOM and secret scans etc)
A->>E: Import SBOM, secret search, fs metadata
E->>E: Scan sbom for vulns and evaluate compliance
Next Steps
Now let’s get familiar with Watching Images and Tags with Anchore.
2.1 - Malware Scanning
Overview
Anchore Enterprise provides malware scanning with the use ClamAV. ClamAV is an open-source antivirus solution designed to detect malicious code embedded in container images.
When enabled, malware scanning occurs with Centralized Analysis, when the image content itself is available.
Any findings are available via:
- API
/images/{image_digest}/content/malware
) - AnchoreCTL
anchorectl image content <image_digest> -t malware
- UI Image SBOM Tab
The Malware Policy Gate also provides compliance rules around any findings.
Please Note: Files in an image which are greater than 2GB will be skipped due to a limitation in ClamAV. Any skipped file will be identified with a Malware Signature as ANCHORE.FILE_SKIPPED.MAX_FILE_SIZE_EXCEEDED
.
Signature DB Updates
Each analyzer service will run a malware signature update before analyzing each image. This does add some latency to the overall analysis time but ensures the signatures
are as up-to-date as possible for each image analyzed. The update behavior can be disabled if you prefer to manage the freshness of the db via another route, such as a shared filesystem
mounted to all analyzer nodes that is updated on a schedule. See the configuration section for details on disabling the db update.
The status of the db update is present in each scan output for each image.
Scan Results
The malware
content type is a list of scan results. Each result is the run of a malware scanner, by default clamav
.
The list of files found to contain malware signature matches is in the findings
property of each scan result. An empty array value indicates no matches found.
The metadata
property provides generic metadata specific to the scanner. For the ClamAV implementation, this includes the version data about the signature db used and
if the db update was enabled during the scan. If the db update is disabled, then the db_version
property of the metadata will not have values since the only way to get
the version metadata is during a db update.
{
"content": [
{
"findings": [
{
"path": "/somebadfile",
"signature": "Unix.Trojan.MSShellcode-40"
},
{
"path": "/somedir/somepath/otherbadfile",
"signature": "Unix.Trojan.MSShellcode-40"
}
],
"metadata": {
"db_update_enabled": true,
"db_version": {
"bytecode": "331",
"daily": "25890",
"main": "59"
}
},
"scanner": "clamav"
}
],
"content_type": "malware",
"imageDigest": "sha256:0eb874fcad5414762a2ca5b2496db5291aad7d3b737700d05e45af43bad3ce4d"
}
3 - Image and Tag Watchers
Overview
Anchore has the capability to monitor external Docker Registries for updates to tags as well as new tags. It also watches for updates to vulnerability databases and package metadata (the “Feeds”).
The process for monitoring updates to repositories, the addition of new tag names, is done on a duty cycle and performed by the Catalog component(s). The scheduling and tasks are driven by queues provided by the SimpleQueue service.
- Periodically, controlled by the cycle_timers configuration in the config.yaml of the catalog, a process is triggered to list all the Repository Subscription records in the system and for each record, add a task to a specific queue.
- Periodically, also controlled by the cycle_timers config, a process is triggered to pick up tasks off that queue and process repository scan tasks. Each task looks approximately like the following:

The output of this process is new tag_update subscription records, which are subsequently processed by the Tag Update handlers as described below. You can view the tag_update subscriptions using AnchoreCTL:
anchorectl subscription list -t tag_update
Tag Updates: New Images
To detect updates to tags, mapping of a new image digest to a tag name, Anchore periodically checks the registry and downloads the tag’s image manifest to compare the computed digests. This is done on a duty cycle for every tag_update subscription record. Therefore, the more subscribed tags exist in the system, the higher the load on the system to check for updates and detect changes. This processing, like repository update monitoring, is performed by the Catalog component(s).
The process, the duty-cycle of which is configured in the cycle_timers section of the catalog config.yaml is described below:

As new updates are discovered, they are automatically submitted to the analyzers, via the image analysis internal queue, for processing.
The overall process and interaction of these duty cycles works like:

Next Steps
Now let’s get familiar with Policy in Anchore.
4 - Analysis Archive
Anchore Enterprise is a data intensive system. Storage consumption grows with the number of images analyzed, which leaves the
following options for storage management:
- Over-provisioning storage significantly
- Increasing capacity over time, resulting in downtime (e.g. stop system, grow the db volume, restart)
- Manually deleting image analysis to free space as needed
In most cases, option 1 only works for a while, which then requires using 2 or 3. Managing storage provisioned for a
postgres DB is somewhat complex and may require significant data copies to new volumes to grow capacity over time.
To help mitigate the storage growth of the db itself, Anchore Enterprise already provides an object storage subsystem that
enables using external object stores like S3 to offload the unstructured data storage needs to systems that are
more growth tolerant and flexible. This lowers the db overhead but does not fundamentally address the issue of unbounded
growth in a busy system.
The Analysis Archive extends the object store even further by providing a system-managed way to move an image analysis
and all of its related data (policy evaluations, tags, annotations, etc) and moving it to a location outside of the main
set of images such that it consumes much less storage in the database when using an object store, perserves the last
state of the image, and supports moving it back into the main image set if it is needed in the future without requiring
that the image itself be reanalzyed–restoring from the archive does not require the actual docker image to exist at all.
To facilitate this, the system can be thought of as two sets of analysis with different capabilities and properties:

Working Set Images
The working set is the set of images in the ‘analyzed’ state in the system. These images are stored in the database,
optionally with some data in an external object store. Specifically:
- State = ‘analyzed’
- The set of images available from the /images api routes
- Available for policy evaluation, content queries, and vulnerability updates
Archive Set Images
The archive set of images are image analyses that reside almost entirely in the object store, which can be configured to
be a different location than the object store used for the working set, with minimal metadata in the anchore DB necessary
to track and restore the analysis back into the working set in the future. An archived image analysis preserves all the
annotations, tags, and metadata of the original analysis as well as all existing policy evaluation histories, but
are not updated with new vulnerabilities during feed syncs and are not available for new policy evaluations or content
queries without first being restored into the working set.
- Not listed in /images API routes
- Cannot have policy evaluations executed
- No vulnerability updates automatically (must be restored to working set first)
- Available from the /archives/images API routes
- Point-in-time snapshot of the analysis, policy evaluation, and vulnerability state of an image
- Independently configurable storage location (analysis_archive property in the services.catalog property of config.yaml)
- Small db storage consumption (if using external object store, only a few small records, bytes)
- Able to use different type of storage for cost effectiveness
- Can be restored to the working set at any time to restore full query and policy capabilities
- The archive object store is not used for any API operations other than the restore process
An image analysis, identified by the digest of the image, may exist in both sets at the same time, they are not mutually
exclusive, however the archive is not automatically updated and must be deleted an re-archived to capture updated state
from the working set image if desired.
Benefits of the Archive
Because archived image analyses are stored in a distinct object store and tracked with their own metadata in the db, the
images in that set will not impact the performance of working set image operations such as API operations, feed syncs, or
notification handling. This helps keep the system responsive and performant in cases where the set of images that you’re
interested in is much smaller than the set of images in the system, but you don’t want to delete the analysis because it
has value for audit or historical reasons.
- Leverage cheaper and more scalable cloud-based storage solutions (e.g. S3 IA class)
- Keep the working set small to manage capacity and api performance
- Ensure the working set is images you actively need to monitor without losing old data by sending it to the archive
Automatic Archiving
To help facilitate data management automatically, Anchore supports rules to define which data to archive and when
based on a few qualities of the image analysis itself. These rules are evaluated periodically by the system.
Anchore supports both account-scoped rules, editable by users in the account, and global system rules, editable only by
the system admin account users. All users can view system global rules such that they can understand what will affect
their images but they cannot update or delete the rules.
The process of automatic rule evaluation:
The catalog component periodically (daily by default, but configurable) will run through each rule in the system and
identify image digests should be archived according to either account-local rules or system global rules.
Each matching image analysis is added to the archive.
Each successfully added analysis is deleted from the working set.
For each digest migrated, a system event log entry is created, indicating that the image digest was moved to the
archive.
Archive Rules
The rules that match images are provide 3 selectors:
- Analysis timestamp - the age of the analysis itself, as expressed in days
- Source metadata (registry, repo, tag) - the values of the registry, repo, and tag values
- Tag history depth – the number of images mapped to a tag ordered by detected_at timestamp (the time at which the
system observed the mapping of a tag to a specific image manifest digest)
Rule scope:
- global - these rules will be evaluated against all images and all tags in the system, regardless of the owning account.
(system_global = true)
- account - these rules are only evaluated against the images and tags of the account which owns the rule. (system_global = false)
Example Rule:
{
"analysis_age_days": 10,
"created_at": "2019-03-30T22:23:50Z",
"last_updated": "2019-03-30T22:23:50Z",
"rule_id": "67b5f8bfde31497a9a67424cf80edf24",
"selector": {
"registry": "*",
"repository": "*",
"tag": "*"
},
"system_global": true,
"tag_versions_newer": 10,
"transition": "archive",
"exclude": {
"expiration_days": -1,
"selector": {
"registry": "docker.io",
"repository": "alpine",
"tag": "latest"
}
},
"max_images_per_account": 1000
}
- selector: a json object defining a set of filters on registry, repository, and tag that this rule will apply to.
- Each entry supports wildcards. e.g.
{"registry": "*", "repository": "library/*", "tag": "latest"}
- tag_versions_newer: the minimum number of tag->digest mappings with newer timestamps that must be preset for this rule to
match an image tag.
- analysis_age_days: the minimum age of the analysis to match, as indicated by the ‘analyzed_at’ timestamp on the image record.
- transition: the operation to perform, one of the following
- archive: works on the working set and transitions to archive, while deleting the source analysis upon successful
archive creation. Specifically: the analysis will “move” to the archive and no longer be in the working set.
- delete: works on the archive set and deletes the archived record on a match
- exclude: a json object defining a set of filters on registry, repository, and tag, that will exclude a subset of image(s)
from the selector defined above.
- expiration_days: This allows the exclusion filter to expire. When set to -1, the exclusion filter does not expire
- max_images_per_account: This setting may only be applied on a single “system_global” rule, and controls the maximum number of images
allows in the anchore deployment (that are not archived). If this number is exceeded, anchore will transition (according to the transition field value)
the oldest images exceeding this maximum count.
- last_seen_in_days: This allows images to be excluded from the archive, if there is a corresponding runtime inventory image, where last_seen_in_days is within the specified number of days.
- This field will exclude any images last seen in X number of days regardless of whether it’s in the exclude selector.
Rule conflicts and application:
For an image to be transitioned by a rule it must:
- Match at least 1 rule for each of its tag entries (either in working set if transition is archive or those in the
archive set, if a delete transition)
- All rule matches must be of the same scope, global and account rules cannot interact
Put another way, if any tag record for an image analysis is not defined to be transitioned, then the analysis record is
not transitioned.
Usage
Image analysis can be archived explicitly via the API (and CLI) as well as restored. Alternatively, the API and CLI can
manage the rules that control automatic transitions. For more information see the following:
Archiving an Image Analysis
See: Archiving an Image
Restoring an Image Analysis
See: Restoring an Image
Managing Archive Rules
See: Working with Archive Rules